SEEK: A Framework of Superpixel Learning with CNN Features for Unsupervised Segmentatio
Published in MDPI Electronics, 2020
Ilyas Talha1, Khan Abbas1, Umraiz Muhammad1, Kim Hyongsuk1,2,*
1Division of Electronic Engineering, Intelligent Robots Research Center, Jeonbuk National University, Jeonju, South Korea
2Division of Electronic and Information Engineering, Jeonbuk National University, Jeonju, South Korea
*Corresponding author: hskim@jbnu.ac.kr
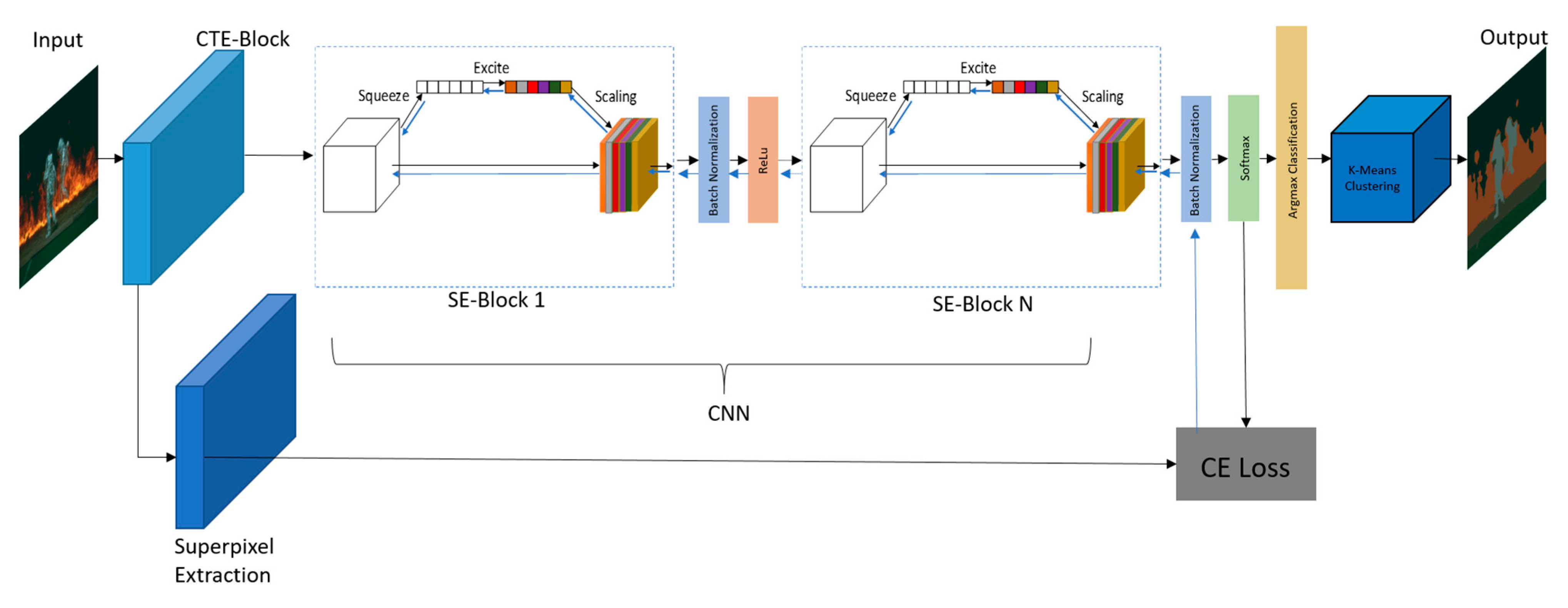
This paper introduces a deep learning based framework for unaided semantic segmentation. The proposed architecture does not need any training data or prior ground truth. It learns to segment the input image by iterating over it repeatedly and assigning specific cluster labels to similar pixels in conjunction, while also updating the parameters of the convolution filters to get even better and more meaningful segmented regions
Abstract
Supervised semantic segmentation algorithms have been a hot area of exploration recently, but now the attention is being drawn towards completely unsupervised semantic segmentation. In an unsupervised framework, neither the targets nor the ground truth labels are provided to the network. That being said, the network is unaware about any class instance or object present in the given data sample. So, we propose a convolutional neural network (CNN) based architecture for unsupervised segmentation. We used the squeeze and excitation network, due to its peculiar ability to capture the features’ interdependencies, which increases the network’s sensitivity to more salient features. We iteratively enable our CNN architecture to learn the target generated by a graph-based segmentation method, while simultaneously preventing our network from falling into the pit of over-segmentation. Along with this CNN architecture, image enhancement and refinement techniques are exploited to improve the segmentation results. Our proposed algorithm produces improved segmented regions that meet the human level segmentation results. In addition, we evaluate our approach using different metrics to show the quantitative outperformance.
Files
Citation
@article{ilyas2020seek,
title={SEEK: A Framework of Superpixel Learning with CNN Features for Unsupervised Segmentation},
author={Ilyas, Talha and Khan, Abbas and Umraiz, Muhammad and Kim, Hyongsuk},
journal={Electronics},
volume={9},
number={3},
pages={383},
year={2020},
publisher={Multidisciplinary Digital Publishing Institute}}